尊敬的用戶您好,這是來自FT中文網的溫馨提示:如您對更多FT中文網的內容感興趣,請在蘋果應用商店或谷歌應用市場搜尋「FT中文網」,下載FT中文網的官方應用。
In January 2020, as doctors struggled to treat the first coronavirus patients, data scientists and biochemists at BenevolentAI turned to artificial intelligence to hunt for a drug to help.
2020年1月,當醫生們在努力治療首批新冠病毒患者時,BenevolentAI的數據科學家和生化學家轉向了人工智慧,尋找一種可以幫助他們的藥物。
The researchers had only a few clues about how the new virus operated. They fed them to their algorithm and set it to work searching 50mn medical journal articles in just a few hours. Together, the scientists and the algorithm deepened their understanding of which biological pathways a drug should target and searched for approved drugs to repurpose.
對於這種新病毒如何運作,研究人員只有很少的線索。他們將這些數據輸入自己的演算法,讓它在短短几小時內搜尋5000萬篇醫學期刊文章。科學家和演算法一起加深了他們對藥物應該針對哪些生物通路的理解,並尋找已批准的藥物來重新利用。
In just four days, they narrowed it down to baricitinib. The Eli Lilly drug usually used to treat rheumatoid arthritis tackles both the virus and the body’s inflammatory reaction. It was the first time AI had discovered a drug to repurpose that has then been used widely.
在短短四天內,他們把範圍縮小到巴利西替尼(baricitinib)。這種美國禮來公司(Eli Lilly)通常用於治療風溼性關節炎的藥物可以同時治療病毒和身體的炎症反應。這是人工智慧首次發現一種可以重新利用的藥物,然後被廣泛使用。
Peter Richardson, the vice-president of pharmacology at the London-based start-up, says AI’s prediction of how the drug would work against Covid — from its biological mechanism to antiviral effect — has now been validated. The World Health Organization strongly recommends the use of baricitinib for severe Covid and the UK’s recovery trial — the national study of treatments for the virus — found it significantly reduces deaths.
這家總部位於倫敦的新創公司的藥理學副總裁彼得·理查森(Peter Richardson)表示,人工智慧對這種藥物如何對抗新冠病毒的預測——從其生物機制到抗病毒效果——現在已經得到了驗證。世界衛生組織強烈建議使用巴利西替尼治療嚴重的新冠肺炎,而英國的康復試驗——一項針對該病毒治療方法的全國性研究——發現它能顯著降低死亡率。
“All of that in two years,” says Richardson. “It is astonishing.”
理查森說:「這一切都在兩年內實現。這是令人驚訝的。」
It takes on average a decade to go from the initial discovery stage of a drug to approval and, with a high failure rate, the pharmaceutical industry estimates it costs $2.7bn to bring each one to market. Clinical trials make up the bulk of this time and cost, but discovery and pre-clinical tests can still take three or four years.
一種藥物從最初的發現階段到獲得批准,平均需要10年時間,而且由於失敗率很高,製藥業估計,每一種藥物的上市成本爲27億美元。臨牀試驗佔據了大部分時間和成本,但發現和臨牀前試驗仍然需要三到四年的時間。


During the pandemic, AI was primarily used as a tool to save scientists’ time, accelerating this notoriously slow discovery process, while often using drugs and vaccines designed for similar viruses such as Mers as a starting point.
在大流行期間,人工智慧主要被用作節省科學家時間的工具,加速這一以緩慢著稱的發現過程,同時經常使用爲類似病毒(如Mers)設計的藥物和疫苗作爲起點。
But advocates of AI believe its wider use during the crisis is just the start of a revolution in drug discovery that will harness growth in biological and chemical data, computing power and smarter algorithms that could reduce soaring healthcare bills and create treatments for conditions where we have none.
但人工智慧的支持者認爲,危機期間人工智慧的廣泛應用,只是一場藥物發現革命的開始。這場革命將利用生物和化學數據、計算能力和更智慧演算法的成長,從而降低不斷飆升的醫療費用,併爲我們的疾病創造先前不存在的治療方法。
They argue AI proved itself in the crisis. AbCellera sorted through 6mn cells in three days to find an antibody that could be mass produced — in this case by Eli Lilly — as a drug called bamlanivimab that has helped more than 1mn Covid patients. A supercomputer helped in Pfizer’s quest for an antiviral that could be taken orally.
他們認爲,人工智慧在這場危機中證明了自己。AbCellera在三天時間裏篩選了600萬個細胞,找到了一種可以大規模生產的抗體——這是由禮來公司生產的——這種名爲bamlanivimab的藥物已經幫助了超過100萬名新冠患者。一臺超級電腦幫助輝瑞公司尋找一種可以口服的抗病毒藥物。
And although AI did not design the Covid vaccines, it optimised them. Moderna’s platform — the technology it uses for all of its vaccines — learnt from producing 20,000 unique mRNA sequences, which helped it design and manufacture the first batch of its Covid vaccine for testing in 42 days.
雖然人工智慧沒有設計Covid疫苗,但它對它們進行了優化。Moderna的平臺——該公司用於所有疫苗的技術——是從生產2萬個獨特的mRNA序列中學習到的,這幫助該公司在42天內設計和製造了第一批用於測試的Covid疫苗。
Venture capitalists are betting this is just the beginning. Funding for AI in drug discovery has risen 3,800 per cent in the past five years to $2bn, according to PitchBook data, and venture capital-backed start-ups have signed dozens of partnerships with Big Pharma.
風險投資家認爲這僅僅是個開始。PitchBook的數據顯示,過去5年,用於人工智慧藥物研發的資金成長了3800倍,達到20億美元,風險資本支援的新創企業已與數十家大型製藥公司簽署了合作伙伴關係。
Nathan Benaich, general partner at Air Street Capital, which invests in AI companies in the life sciences field, says the pandemic has reinforced the idea that drugmakers need to speed up the discovery process. “The clear benefit coming out of all this is that AI companies in this field are now pushing at much more of an open door in pharma versus before because of the obvious need for rapid innovation,” he says.
投資生命科學領域人工智慧公司的Air Street Capital普通合夥人內森•貝奈奇(Nathan Benaich)表示,大流行強化了製藥商需要加快發現過程的想法。他表示:「這一切帶來的明顯好處是,由於對快速創新的明顯需求,該領域的人工智慧公司現在正在向製藥行業推進一扇更加開放的大門。」
But even Benaich thinks it has not lived up to the inflated hopes at the start of the pandemic — and he is far from alone.
但是,就連貝奈奇也認爲,這並沒有達到大流行開始時人們膨脹的期望——而且他並不是唯一這樣認爲的人。
“I think repurposing [of drugs] is still worth pursuing,” says Eric Topol, author of Deep Medicine, a book on artificial intelligence in healthcare. “But we don’t have a lot to show for it. In the pandemic, we have one drug for sure . . . but the whole idea of AI was to take drugs and go into discovery at high velocities like we’ve never seen before.”
《深度醫學》(Deep Medicine)是一本關於醫療領域人工智慧的書,該書的作者埃裏克•託波爾(Eric Topol)表示:「我認爲(藥物的)再利用仍然值得追求。但我們沒有很多東西可以展示。在大流行中,我們肯定有一種藥…但人工智慧的整體理念是找到藥物,並以我們從未見過的高速度進行發現。」
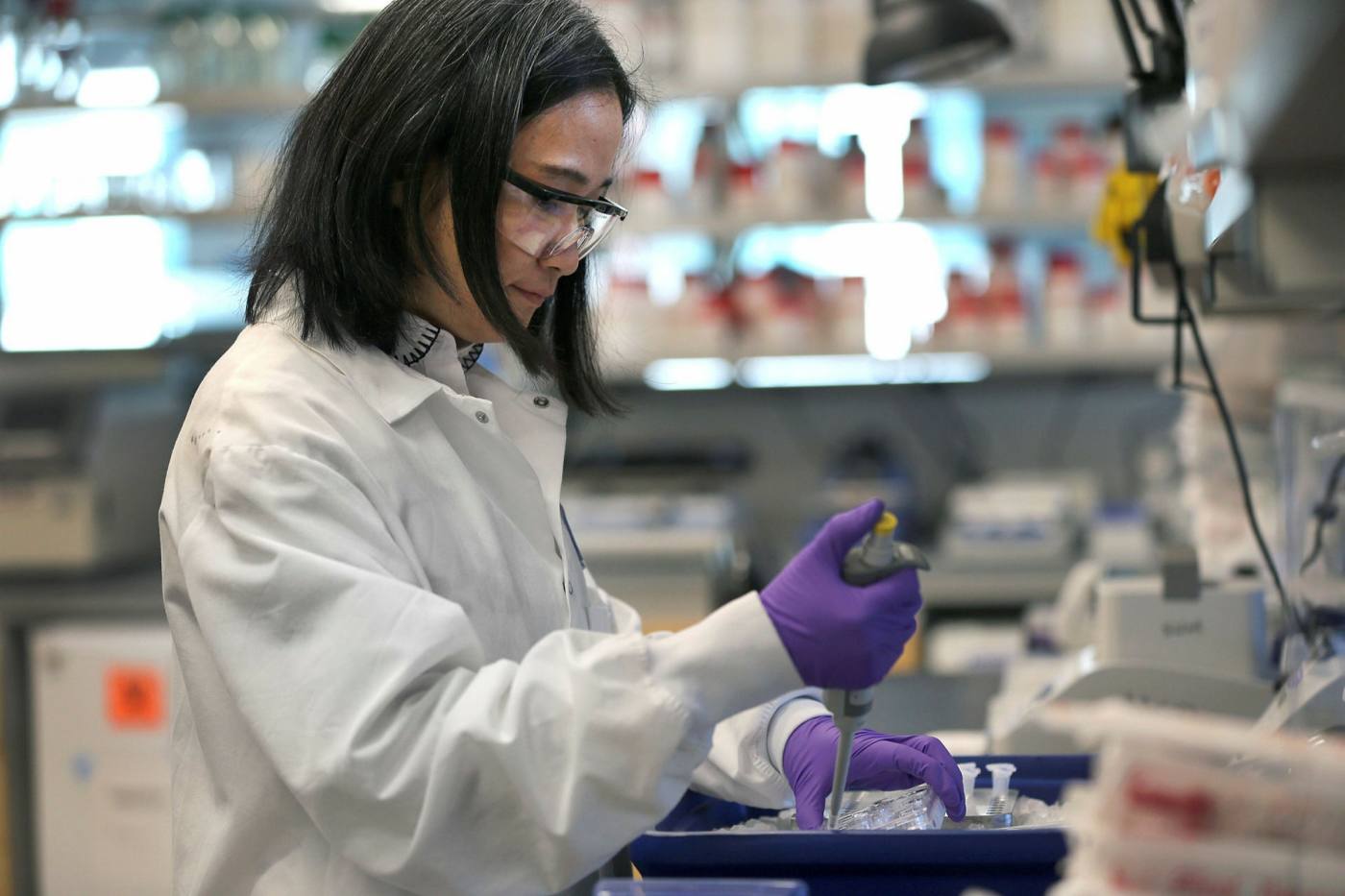
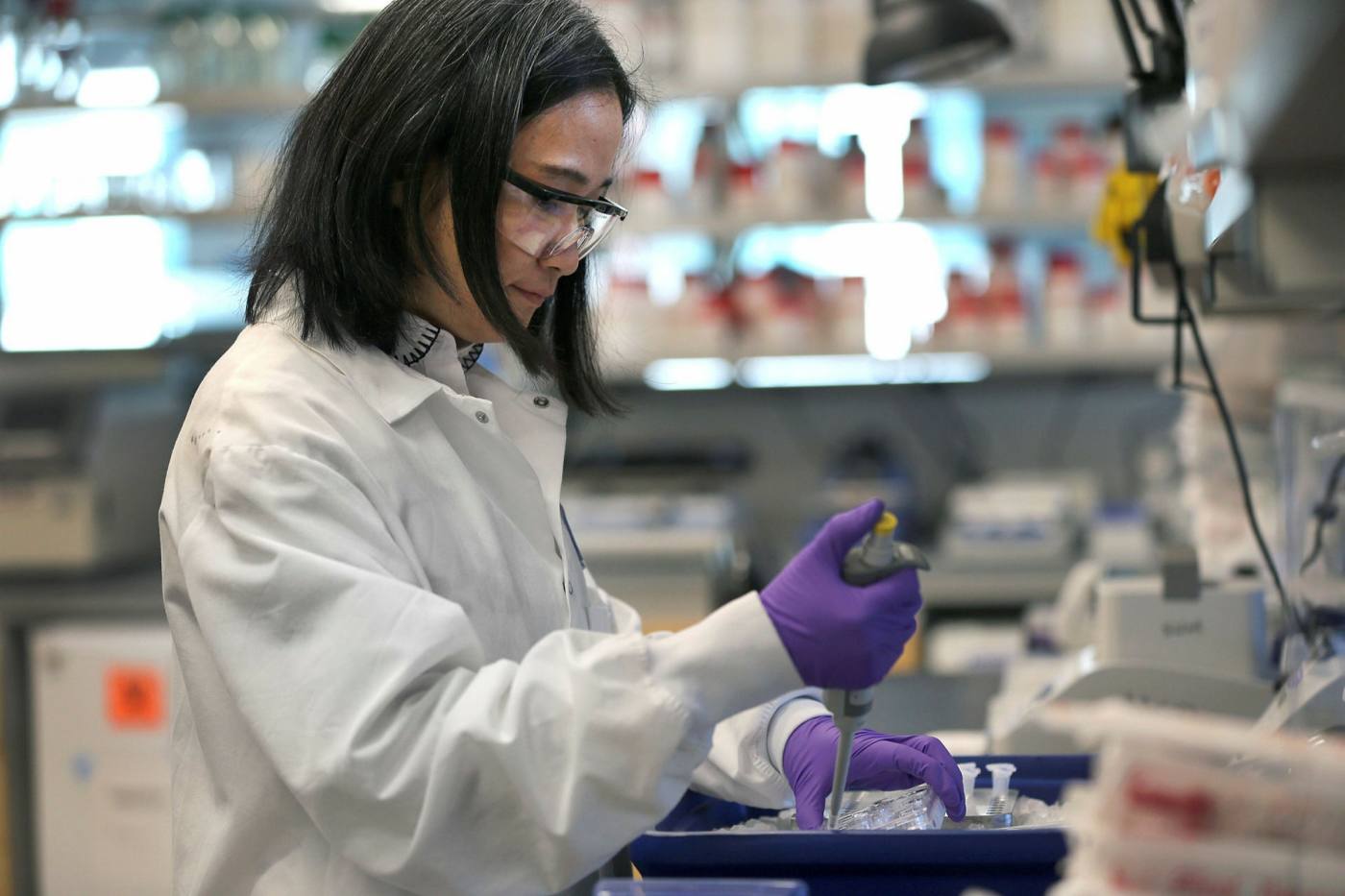
Until a regulator approves a drug discovered entirely by AI, there will be doubters. There are now 15 drug candidates designed by AI in clinical trials — but precisely because drug development can take more than a decade, it will be a long wait for a verdict.
在監管機構批准完全由人工智慧發現的藥物之前,肯定會有人持懷疑態度。目前有15種由人工智慧設計的候選藥物正在進行臨牀試驗,但正是因爲藥物開發可能需要10年以上的時間,所以要等待結果將是一個漫長的過程。
“There’s still an awful lot of people”, says Richardson, “that don’t believe a word of it and think it’s all hype.”
理查森說:「仍然有相當多的人不相信,認爲這都是炒作。」
Safety valves
安全閥
As the pandemic progressed, clinicians around the world looked to recommendations from the UK’s recovery trial to guide their prescriptions. Martin Landray, a professor of medicine and epidemiology at Oxford university, helped run the randomised controlled trial for Covid drugs. But when selecting what to test in patients, he says, he found AI only “moderately useful”.
隨著疫情的發展,世界各地的臨牀醫生都在尋求英國康復試驗的建議,以指導他們的處方。牛津大學醫學和流行病學教授馬丁·蘭德雷(Martin Landray)幫助開展了Covid藥物的隨機對照試驗。但他說,在選擇在病人身上測試什麼時,他發現人工智慧「用處不大」。
“The trouble is [the drug discovery algorithms] all come up with a prioritised list [of treatments] in a different order than you put in,” he says, adding the “mathematical rationale” often fails to consider practicalities.
他表示:「問題是(藥物發現演算法)都會按照不同的順序列出(治療方法)的優先順序。」他補充稱,「數學原理」往往沒有考慮到實用性。
The recovery trial’s investigators chose drugs based on having some evidence that they might work — such as tackling previous coronaviruses or proving effective in pre-clinical tests. But they also took into account whether the drug was in plentiful supply, and how much was understood about side effects.
康復試驗的調查人員選擇藥物的依據是有一些證據表明它們可能有效,比如應對之前的新冠病毒或在臨牀前測試中證明有效。但他們也考慮了該藥物是否供應充足,以及對其副作用的瞭解程度。
Landray says safety has almost “infinite dimensions” that AI struggles to predict, far more complex than some of its biggest successes, such as learning to beat humans at the ancient Chinese game Go. “Instead of trying to play Go, [AI in drug discovery] is like trying to play every game that has ever been invented, some of which don’t even have rules,” he says.
蘭德雷表示,安全幾乎具有人工智慧難以預測的「無限維度」,遠比人工智慧取得的一些最大成功要複雜得多,比如在古老的中國遊戲圍棋中學會擊敗人類。他表示:「(人工智慧在藥物研發中的應用)不是試圖玩圍棋,而是試圖玩所有被髮明出來的遊戲,其中有些甚至沒有規則。」
Let loose on the world of biology, AI could also be dangerous. Researchers recently showed how their algorithm discovered 40,000 toxic chemical compounds, many of which could be used as biological weapons, in less than six hours.
在生物世界中,人工智慧也可能是危險的。研究人員最近展示了他們的演算法是如何在不到6小時的時間裏發現4萬種有毒化合物的,其中許多可以被用作生物武器。
Covid-19 showed the need for rapid drug development — but the initial lack of information about the disease meant it was not the perfect proof point for AI. At the start, there was little data for an algorithm to learn from, meaning even Benevolent’s scientists had to take a more hands-on approach — using AI as an interactive tool — than they would with other diseases.
Covid-19表明需要快速開發藥物,但最初缺乏有關這種疾病的資訊,這意味著它不是人工智慧的完美證明點。一開始,可供演算法學習的數據很少,這意味著即使是Benevolent的科學家也不得不採取一種更親力親爲的方法——將人工智慧作爲一種互動工具——而不是像對待其他疾病那樣。


Daphne Koller, chief executive of Silicon Valley based AI start-up Insitro, which focuses on drug discovery, applauds Benevolent for demonstrating that it is feasible to find a drug to repurpose, but warns that it is different from discovering a completely new compound.
位於矽谷的人工智慧新創公司Insitro的首席執行長達芙妮•科勒(Daphne Koller)專注於藥物發現,她稱讚Benevolent公司證明了找到一種可以重新利用的藥物是可行的,但她警告說這與發現一種全新的化合物不同。
“I’m not a fan of going out with extravagant promises about how we’re going to transform everything within three years,” she says. “Human biology is hard: intervening in something that can cause someone to die is a very risky proposition.”
她說:「我不喜歡隨便許下什麼我們將在三年內改變一切的奢侈承諾。人類生物學很難:干預可能導致人死亡的事情是一個非常危險的命題。」
Another similarly named AI start-up Insilico designed its own Covid drug. The Hong Kong-based company published a paper on its findings in April 2020 for anyone to use. When no one decided to develop it, the company synthesised the drug itself in September of the same year. But without the facilities of a large pharmaceutical company, it had to wait nine months to test it in a lab secure enough to handle the virus. And it is still yet to reach human trials.
另一家名字相似的人工智慧新創公司Insilico設計了自己的Covid藥物。這家位於香港的公司在2020年4月發表了一篇關於其發現的論文,供任何人使用。當沒有人決定開發它時,該公司在同年9月自己合成了這種藥物。但由於沒有大型製藥公司的設施,它不得不等待9個月,在一個足以處理病毒的安全實驗室進行測試。而且它仍未進入人體試驗階段。
The pandemic highlighted the two biggest hurdles for the future of AI in drug discovery: making sense of the patchy but ever more plentiful data, and changing the strategies of large pharmaceutical companies cautious about getting into bed with AI.
這場大流行凸顯了人工智慧在藥物發現領域未來的兩個最大障礙:使零散但日益豐富的數據變得有意義,以及改變對與人工智慧合作持謹慎態度的大型製藥公司的策略。
Frank Nestlé, Sanofi’s chief scientific officer, is embracing AI with vigour, having recently signed two big deals with Oxford-based Exscientia and medical AI company Owkin. He says AI relies on three pillars — computing power, algorithms and data.
賽諾菲(Sanofi)首席科學官弗蘭克·內斯特爾(Frank Nestlé)正積極擁抱人工智慧。最近,他與總部位於牛津的Exscientia和醫療人工智慧公司Owkin簽署了兩項重大協議。他說,人工智慧依賴三大支柱——計算能力、演算法和數據。
“Data is where there’s the biggest need for improvement — and that’s also where the future step change will come,” he adds.
他補充道:「數據是最需要改進的地方,也是未來需要改變的地方。」
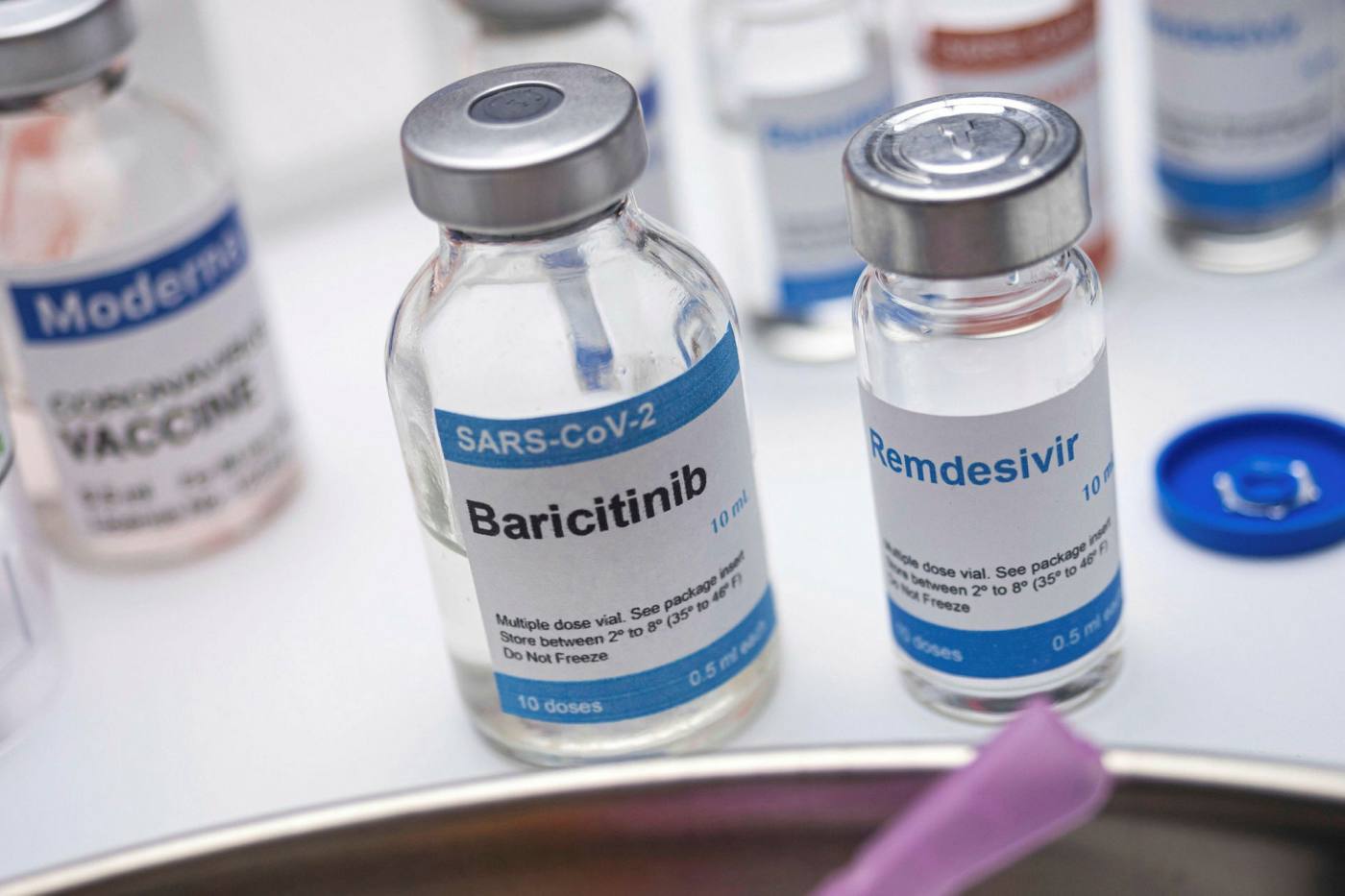
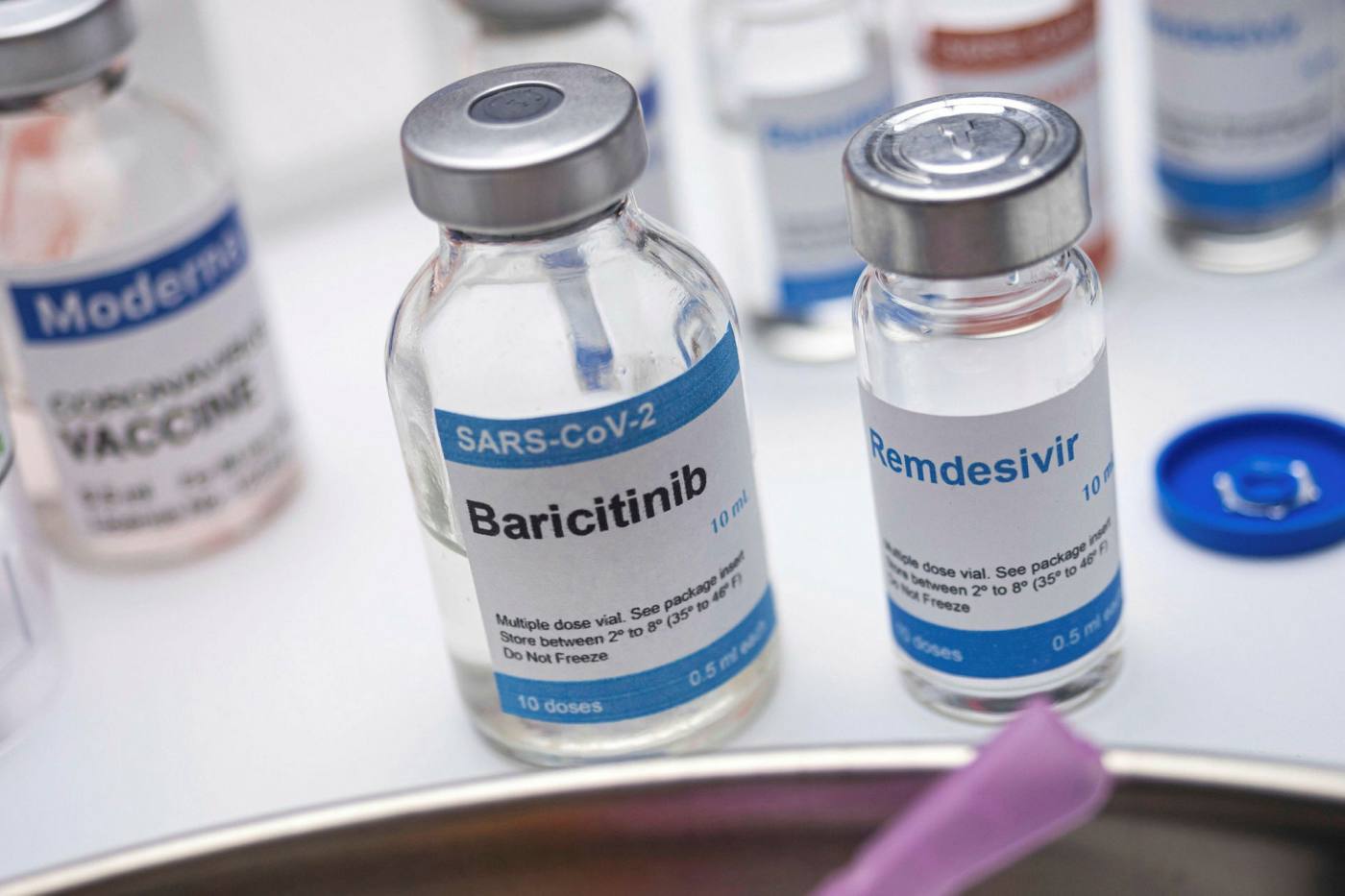
There has been an explosion of data because it has become much easier and cheaper to collect sequences of genes and proteins. Drugmakers hold huge stores of data from trials on humans and animals, but it is often in forms AI finds difficult to digest, from handwritten lab notes to Excel spreadsheets.
由於收集基因和蛋白質的序列變得更加容易和便宜,數據已經出現了爆炸。製藥商擁有大量來自人類和動物試驗的數據,但這些數據往往是人工智慧難以消化的形式,從手寫的實驗筆記到Excel電子表格。
Nestlé says tagging this data requires painstaking precision: for example, lab samples shipped overnight should be differentiated from others, because they have different molecular footprints that could drive algorithms to the wrong conclusions based on how it was shipped, not what was in the patient’s tissue.
內斯特爾表示,標記這些數據需要艱苦的精確性:例如,隔夜運送的實驗室樣本應與其他樣本區分開來,因爲它們有不同的分子足跡,可能會促使演算法根據運送方式而不是病人組織中的內容得出錯誤結論。
Such work takes time. Ugur Sahin, BioNTech chief executive, says the German biotech used a “man-in-the-middle” approach to machine learning for four years with humans helping to educate its algorithm. “AI is like kids. You have to really teach them a lot until they start to come to conclusions,” he says.
這樣的工作需要時間。BioNTech首席執行長烏格•沙欣(Ugur Sahin)表示,這家德國生物技術公司在4年時間裏使用了一種「中間人」的方法來進行機器學習,由人類幫助培訓演算法。「人工智慧就像孩子。你必須教他們很多東西,直到他們開始得出結論,」他說。
Companies that invested in their data have seen it pay dividends. In 2020, Pfizer could not automatically screen one of its libraries that holds data on 4.5bn commercially available compounds. Now, it can scan the entire database in 48 hours vastly speeding up its ability to identify potential new medicines.
對數據進行投資的公司已經看到了回報。2020年,輝瑞(Pfizer)無法自動篩選其擁有45億種商業可用化合物數據的資料庫之一。現在,它可以在48小時內掃描整個資料庫,大大加快了它識別潛在新藥的能力。
Others stress the importance of hiring employees who can bridge the gap between AI and more traditional scientific methods. Najat Khan, chief data science officer and head of R&D strategy at Janssen, the pharmaceuticals arm of Johnson & Johnson, says it is building a bank of “bilingual talent”.
另一些人則強調僱用能夠在人工智慧和更傳統的科學方法之間架起橋樑的員工的重要性。嬌生(Johnson & Johnson)的製藥部門楊森(Janssen)的首席數據科學官兼研發戰略主管納賈特·汗(Najat Khan)說,它正在建立一個「雙語人才」庫。
“We have data scientists who are highly proficient in machine learning, in AI, in digital health and real world evidence — and deeply knowledgeable in drug discovery science and medicine,” she adds.
她補充說:「我們的數據科學家精通機器學習、人工智慧、數字健康和真實世界證據,並且對藥物發現科學和醫學有深刻的瞭解。」
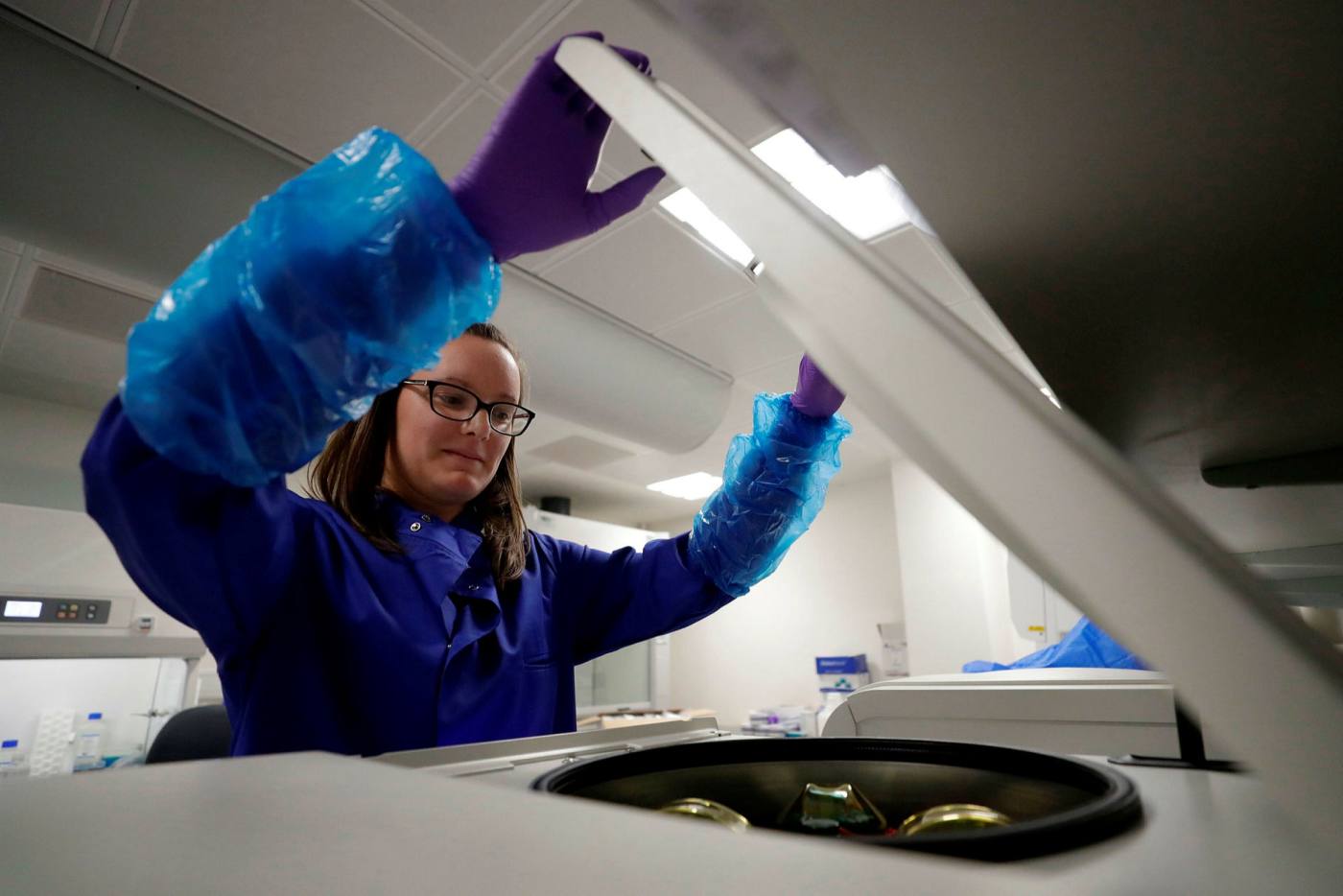
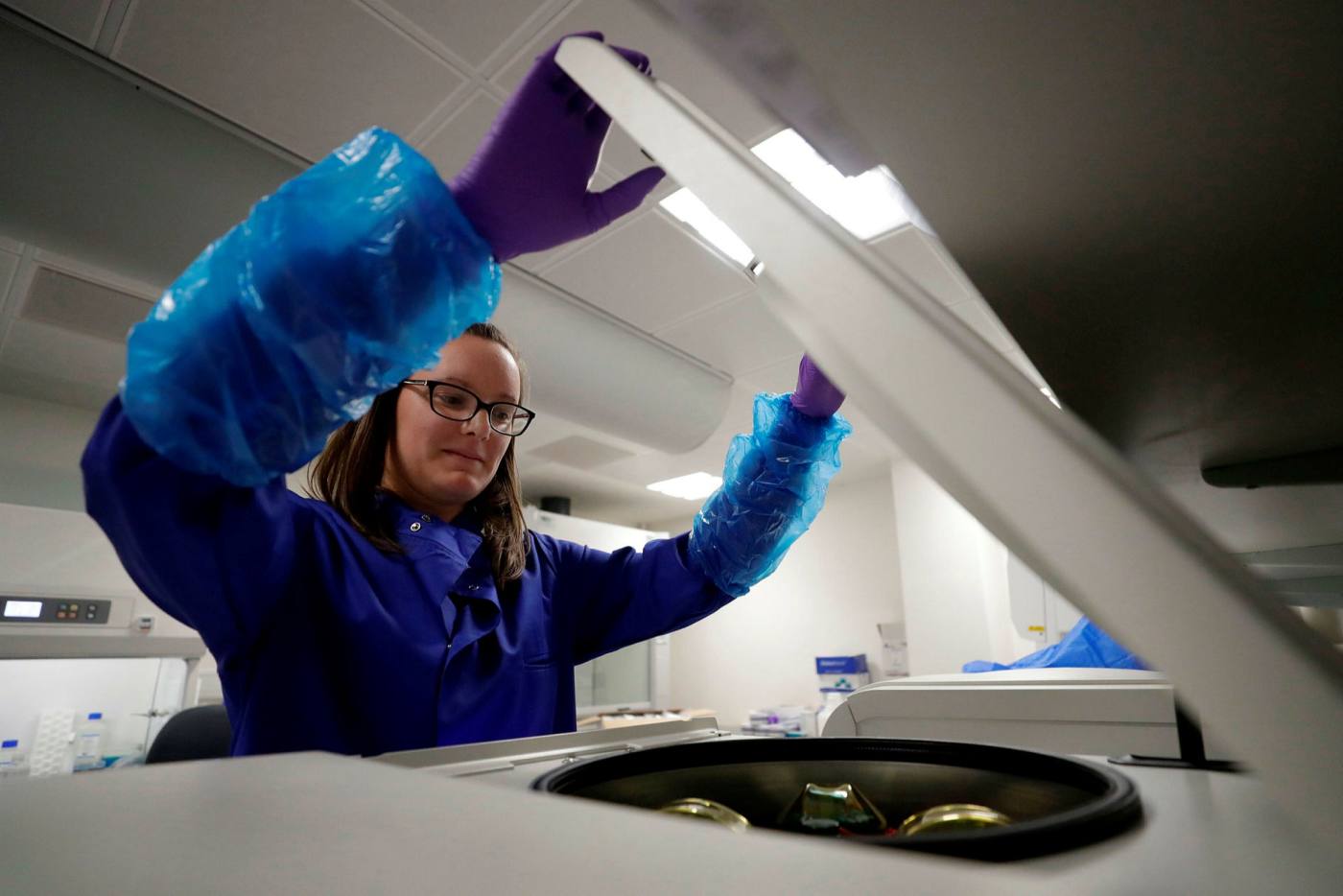
But some pharmaceutical companies are still figuring out how best to incorporate AI, unsure about how much to invest in the new field rather than progressing their current pipelines and struggling to hire talent from technology companies.
但一些製藥公司仍在摸索如何最好地納入人工智慧,不確定應該在新領域投資多少,以及應該在花多大力氣繼續推進他們目前的管道,並難以從技術公司招聘人才。
Miraz Rahman, a professor of medicinal chemistry at King’s College London, says the industry has been burnt before by pouring money into combinatorial chemistry, a method of building libraries of compounds that has been useful in recent decades but not as transformative as promised.
倫敦大學國王學院藥物化學教授米拉茲·拉赫曼(Miraz Rahman)說,該行業曾經因爲向組合化學傾注資金而被遭受重挫,這種建立化合物庫的方法在最近幾十年裏一直很有用,但並不像承諾的那樣具有變革性。
Yet he believes that in the next five to 10 years, all big pharma companies will have integrated AI into drug discovery. “All the data suggests AI is here to stay,” adds Rahman. “It is going to make discovery more streamlined and shave off three to four years of time if you can successfully adopt AI.”
但他認爲,在未來5至10年內,所有大型製藥公司都將把人工智慧整合到藥物研發中。拉赫曼補充道:「所有數據都表明,人工智慧將繼續存在。如果你能成功採用人工智慧,它將使發現變得更流暢,並節省三到四年的時間。」
Unlocking science
解鎖科學
Thomas Clozel, chief executive of Owkin, says repurposing drugs is simply a “hack” and that the long-term hope for AI is that it can transform our understanding of disease.
Owkin首席執行長托馬斯•克洛澤爾(Thomas Clozel)表示,將藥物重新利用只是一種「修修補補」,人工智慧的長期希望在於,它可以改變我們對疾病的理解。
“AI today isn’t ready to treat patients better. It is ready to accelerate the return on investment of drug discovery,” he says. “But what about augmenting it — when are you going to find things where pharma failed?”
「今天的人工智慧還沒有準備好更好地治療病人。它已準備好加速藥物研發的投資回報,」他說。「但如何擴大它呢?你什麼時候才能發現製藥公司失敗的地方?」
He thinks the real innovation will come from empirical evidence mined from “patient data”. Owkin builds data sets from hospitals and analyses cellular level information to find new patient subgroups. Using computer vision — AI that can understand and read images — to study biopsies, the company can predict survival rates in patients with the aggressive cancer mesothelioma, which mainly affects the lining of the lungs. This could unlock doors for drugmakers to compounds, which might increase survival rates.
他認爲,真正的創新將來自於從「患者數據」中挖掘出的實證證據。Owkin建立了來自醫院的數據集,並分析細胞層面的資訊,以發現新的患者亞羣。該公司利用電腦視覺(一種能夠理解和讀取影像的人工智慧)來研究活組織檢查,可以預測侵襲性腫瘤間皮瘤患者的存活率。間皮瘤主要影響肺部粘膜。這可能會爲製藥商打開開發複合藥物的大門,從而提高存活率。
The deeper our understanding, the more personalised medicine may become. Before it became famous for its Covid vaccine, BioNTech was focused on creating “cancer vaccines” tailored to direct an individuals’ immune system to attack his or her own disease, using data from an extracted tumour. It is now ploughing ahead with that work and with AI at its core.
我們理解得越深,藥物的個性化程度可能就越高。在因新冠病毒疫苗而出名之前,BioNTech專注於開發「癌症疫苗」,利用提取的腫瘤數據,定製個性化的「癌症疫苗」,引導個人的免疫系統攻擊他或她自己的疾病。目前,該公司正以人工智慧爲核心,努力推進這項工作。
“The question is: if every patient has different mutations, could we build machine learning programmes that allow us to pick the best targets in each patient?” asks Sahin.
「問題是:如果每個患者都有不同的突變,我們能否構建機器學習程式,讓我們能夠在每個患者身上選擇最佳目標?」沙欣問道。
It is an “extremely complex task”, he adds, that involves weighing factors including the likelihood a mutation is in the tumour and the probability that the immune system’s T-cells recognise it. But he adds that machine learning has become “better and better”, and now humans only intervene for quality assurance.
他補充說,這是一項「極其複雜的任務」,涉及到權衡因素,包括腫瘤發生突變的可能性和免疫系統的T細胞識別突變的可能性。但他補充說,機器學習已經變得「越來越好」,現在人類干預只是爲了保證質量。


Other innovations may accelerate progress. Last year, Google’s DeepMind released an AI tool called Alphafold2 that can predict the shape of all human proteins. By solving this huge puzzle in biology, it helps scientists understand what each protein does and how to alter it. Drugmakers can use it to understand where a drug might need to bind to a target in a human body. DeepMind plans to do just that, launching a new company Isomorphic Labs to “find cures for humanity’s most devastating diseases”.
其他創新可能會加速進步。去年,谷歌的DeepMind發佈了一款名爲Alphafold2的人工智慧工具,它可以預測所有人類蛋白質的形狀。透過解決這個生物學上的巨大難題,它有助於科學家瞭解每種蛋白質的作用以及如何改變它。製藥商可以利用它來了解藥物可能需要在人體的哪個部位與目標結合。DeepMind正計劃這樣做,成立一家新公司同構實驗室(Isomorphic Labs),以「找到治療人類最具破壞性疾病的方法」。
Large pharmaceutical companies are interested in using the tool. J&J’s Khan says there are so many proteins that are considered “undruggable” because of a tricky protein. “It is going to unlock a lot of science that was impossible and make it possible,” she says.
大型製藥公司對使用這個工具很感興趣。嬌生公司的汗說,由於一種棘手的蛋白質,有很多蛋白質被認爲是「無藥可治」的。她說:「這將解開許多以前不可能的科學之謎,並使之成爲可能。」
Advances in quantum computing could also massively increase the available computer power to help tackle problems in drug discovery. But for now, AI may prove more helpful with the next generation of Covid drugs and vaccines.
量子計算的進步也可以大大增加可用的電腦能力,以幫助解決藥物發現方面的問題。但就目前而言,人工智慧可能對下一代抗疫藥物和疫苗更有幫助。
The virus is now one of the most scrutinised ever. BioNTech has partnered with AI start-up InstaDeep to predict the next high-risk variant, so its flexible mRNA vaccine platform can get ahead of it. The resultant algorithm identified more than 90 per cent of variants of concern, including the Omicron strain, in advance. AbCellera used AI to select the most robust antibody that can stand up better to variants and Exscientia wants to create a broad spectrum antiviral that could be used to tackle the next pandemic virus, whatever it turns out to be.
這種病毒現在是有史以來最受關注的病毒之一。BioNTech已與人工智慧新創公司InstaDeep合作,預測下一個高風險變異,因此其靈活的mRNA疫苗平臺可以領先於它。由此產生的演算法提前識別出了90%以上的擔憂變種,包括奧密克戎(Omicron)毒株。AbCellera利用人工智慧選擇了最強大的抗體,這種抗體能夠更好地抵禦變異,而Exscientia希望創造一種廣譜抗病毒藥物,可以用來對付下一種大流行病毒,無論它最終會是什麼。
Andrew Hopkins, Exscientia chief executive, says he is starting to see a “sea change” as larger pharmaceutical companies are more interested in investing in AI.
Exscientia首席執行長安德魯•霍普金斯(Andrew Hopkins)表示,隨著大型製藥公司對投資人工智慧更感興趣,他開始看到一種「鉅變」。
“There is a realisation of how we can use data and algorithms,” he says. “That what the industry did for coronavirus patients is actually something we should apply for all patients. It’s an urgency that we should bring to all medicines for all patients.”
他表示:「我們已經認識到了如何利用數據和演算法。該行業爲新冠病毒患者所做的,實際上我們應該適用於所有患者。我們迫切需要爲所有患者帶來所有藥物。」
Additional reporting by Madhumita Murgia in London
Madhumita Murgia倫敦補充報導